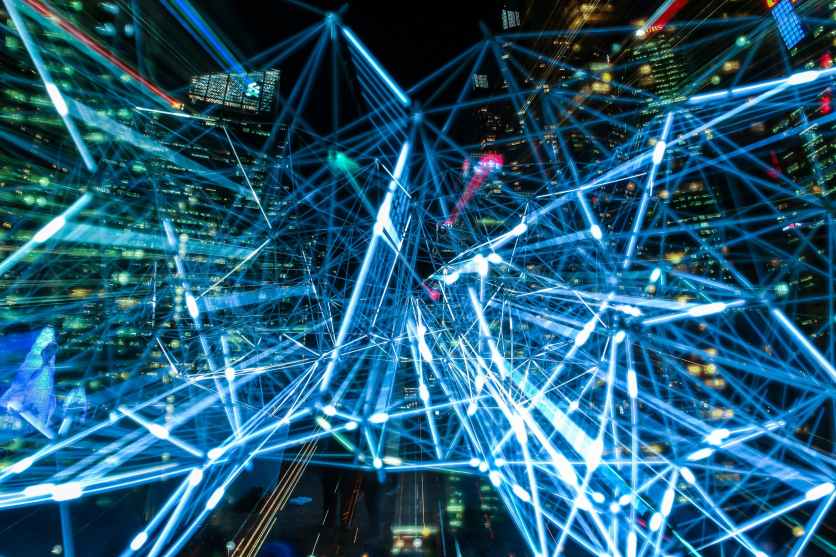
Pixabay/Pexels
This story was originally published by Yale E360 and is reproduced here as part of the Climate Desk collaboration.
Two months after its release in November 2022, OpenAI’s ChatGPT had 100 million active users, and suddenly tech corporations were racing to offer the public more “generative AI” Pundits compared the new technology’s impact to the Internet, or electrification, or the Industrial Revolution—or the discovery of fire.
Time will sort hype from reality, but one consequence of the explosion of artificial intelligence is clear: this technology’s environmental footprint is large and growing.
AI use is directly responsible for carbon emissions from non-renewable electricity and for the consumption of millions of gallons of fresh water, and it indirectly boosts impacts from building and maintaining the power-hungry equipment on which AI runs. As tech companies seek to embed high-intensity AI into everything from resume-writing to kidney transplant medicine and from choosing dog food to climate modeling, they cite many ways AI could help reduce humanity’s environmental footprint. But legislators, regulators, activists, and international organizations now want to make sure the benefits aren’t outweighed by AI’s mounting hazards.
“The development of the next generation of AI tools cannot come at the expense of the health of our planet,” Massachusetts Sen. Edward Markey (D) said last week in Washington, after he and other senators and representatives introduced a bill that would require the federal government to assess AI’s current environmental footprint and develop a standardized system for reporting future impacts. Similarly, the European Union’s “AI Act,” approved by member states last week, will require “high-risk AI systems” (which include the powerful “foundation models” that power ChatGPT and similar AIs) to report their energy consumption, resource use, and other impacts throughout their systems’ lifecycle. The EU law takes effect next year.
Meanwhile, the International Organization for Standardization, a global network that develops standards for manufacturers, regulators, and others, says it will issue criteria for “sustainable AI” later this year. Those will include standards for measuring energy efficiency, raw material use, transportation, and water consumption, as well as practices for reducing AI impacts throughout its life cycle, from the process of mining materials and making computer components to the electricity consumed by its calculations. The ISO wants to enable AI users to make informed decisions about their AI consumption.
Right now, it’s not possible to tell how your AI request for homework help or a picture of an astronaut riding a horse will affect carbon emissions or freshwater stocks. This is why 2024’s crop of “sustainable AI” proposals describe ways to get more information about AI impacts.
In the absence of standards and regulations, tech companies have been reporting whatever they choose, however they choose, about their AI impact, says Shaolei Ren, an associate professor of electrical and computer engineering at UC Riverside, who has been studying the water costs of computation for the past decade. Working from calculations of annual use of water for cooling systems by Microsoft, Ren estimates that a person who engages in a session of questions and answers with GPT-3 (roughly 10 t0 50 responses) drives the consumption of a half-liter of fresh water. “It will vary by region, and with a bigger AI, it could be more.”
But a great deal remains unrevealed about the millions of gallons of water used to cool computers running AI, he says. The same is true of carbon.
“Data scientists today do not have easy or reliable access to measurements of [greenhouse gas impacts from AI], which precludes development of actionable tactics,” a group of 10 prominent researchers on AI impacts wrote in a 2022 conference paper. Since they presented their article, AI applications and users have proliferated, but the public is still in the dark about those data, says Jesse Dodge, a research scientist at the Allen Institute for Artificial Intelligence in Seattle, who was one of the paper’s coauthors.
AI can run on many devices—the simple AI that autocorrects text messages will run on a smartphone. But the kind of AI people most want to use is too big for most personal devices, Dodge says. “The models that are able to write a poem for you, or draft an email, those are very large,” he says. “Size is vital for them to have those capabilities.”
Big AIs need to run immense numbers of calculations very quickly, usually on specialized Graphical Processing Units—processors originally designed for intense computation to render graphics on computer screens. Compared to other chips, GPUs are more energy-efficient for AI, and they’re most efficient when they’re run in large “cloud data centers”—specialized buildings full of computers equipped with those chips. The larger the data center, the more energy efficient it can be. Improvements in AI’s energy efficiency in recent years are partly due to the construction of more “hyperscale data centers,” which contain many more computers and can quickly scale up. Where a typical cloud data center occupies about 100,000 square feet, a hyperscale center can be 1 or even 2 million square feet.
Estimates of the number of cloud data centers worldwide range from around 9,000 to nearly 11,000. More are under construction. The International Energy Agency (IEA) projects that data centers’ electricity consumption in 2026 will be double that of 2022—1,000 terawatts, roughly equivalent to Japan’s current total consumption.
However, as an illustration of one problem with the way AI impacts are measured, that IEA estimate includes all data center activity, which extends beyond AI to many aspects of modern life. Running Amazon’s store interface, serving up Apple TV’s videos, storing millions of people’s emails on Gmail, and “mining” Bitcoin are also performed by data centers. (Other IEA reports exclude crypto operations, but still lump all other data-center activity together.)